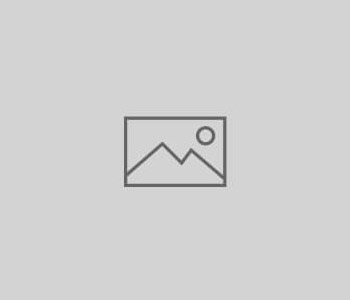
Ground-breaking paper on Integrative Causal Analysis published in JMLR
Is it possible to predict a disease is correlated with an exposure factor without ever performing a study to measure them? The answer is shown to be yes, in some cases, by analyzing existing datasets that measure these two quantities, but not jointly. The idea is to induce all causal models that are simultaneously consistent with several existing datasets and make novel statistical inferences, sometimes regarding quantities never jointly measured together. We have named the approach Integrative Causal Analysis (INCA). A recent important publication in the Journal of Machine Learning Research shows that INCA often makes new and very accurate inferences. It works ubiquitously on all domains we have tried it. It is strong evidence that theories of Computational Causal Discovery can go further than classical statistics: modeling and inducing causal relations gives more inferences capabilities.